Journal Article: 'A novel spatio-temporal clustering algorithm with applications on COVID-19 data from the United States' - Prof. Soudeep Deb
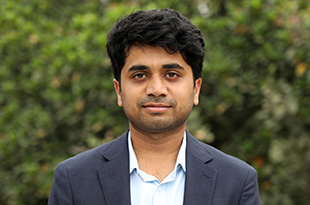
Abstract: A new clustering algorithm for spatio-temporal data is developed. The proposed method leverages a weighted combination of a spatial haversine distance matrix and a spectral-density based temporal distance matrix between the locations. Concepts of partition around medoids algorithm and the gap statistic are utilized to develop the algorithm and to determine the optimal number of clusters. Such a non-parametric algorithm is novel as it incorporates both spatial and temporal distances of the units and it can work for time-series of possibly different lengths. Theoretical guarantee of consistency of the proposed method is provided. An elaborate simulation study is also given to demonstrate the efficacy of the algorithm. As an interesting real life application, the proposed algorithm is implemented to analyze the spatio-temporal dynamics of the time series of coronavirus (COVID-19) incidence rates observed at county-level in the United States of America. The results are demonstrated on datasets of different sizes: the entire country, the Midwest region and the state of California. Special emphasis is given on the last two cases to display how the clustering results offer interesting insights into the epidemic progression in these areas. Particularly, it sheds light on whether state-mandated restrictions impacted the entire state similarly or if there are interesting local behaviors in terms of the COVID-19 spread.
Authors’ Names: Soudeep Deb and Sayar Karmakar
Journal Name: Computational Statistics & Data Analysis
URL: https://www.sciencedirect.com/science/article/pii/S0167947323001214
Journal Article: 'A novel spatio-temporal clustering algorithm with applications on COVID-19 data from the United States' - Prof. Soudeep Deb
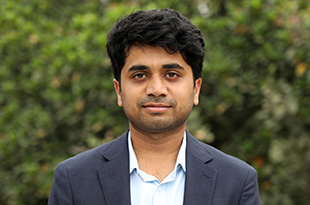
Abstract: A new clustering algorithm for spatio-temporal data is developed. The proposed method leverages a weighted combination of a spatial haversine distance matrix and a spectral-density based temporal distance matrix between the locations. Concepts of partition around medoids algorithm and the gap statistic are utilized to develop the algorithm and to determine the optimal number of clusters. Such a non-parametric algorithm is novel as it incorporates both spatial and temporal distances of the units and it can work for time-series of possibly different lengths. Theoretical guarantee of consistency of the proposed method is provided. An elaborate simulation study is also given to demonstrate the efficacy of the algorithm. As an interesting real life application, the proposed algorithm is implemented to analyze the spatio-temporal dynamics of the time series of coronavirus (COVID-19) incidence rates observed at county-level in the United States of America. The results are demonstrated on datasets of different sizes: the entire country, the Midwest region and the state of California. Special emphasis is given on the last two cases to display how the clustering results offer interesting insights into the epidemic progression in these areas. Particularly, it sheds light on whether state-mandated restrictions impacted the entire state similarly or if there are interesting local behaviors in terms of the COVID-19 spread.
Authors’ Names: Soudeep Deb and Sayar Karmakar
Journal Name: Computational Statistics & Data Analysis
URL: https://www.sciencedirect.com/science/article/pii/S0167947323001214